In medical image segmentation tasks, deep learning-based models usually require densely and precisely annotated datasets to train, which are time-consuming and expensive to prepare. One possible solution is to train with the mixed-supervised dataset, where only a part of data is densely annotated with segmentation map and the rest is annotated with some weak form, such as bounding box. In this work, we propose a novel network architecture called Mixed-Supervised Dual-Network (MSDN), which consists of two separate networks for the segmentation and detection tasks respectively, and a series of connection modules between the layers of the two networks. These connection modules are used to extract and transfer useful information from the detection task to help the segmentation task. We exploit a variant of a recently designed technique called ‘Squeeze and Excitation’ in the connection module to boost the information transfer between the two tasks. Compared with existing model with shared backbone and multiple branches, our model has flexible and trainable feature sharing fashion and thus is more effective and stable.

Classifying ground-glass lung nodules (GGNs) into atypical adenomatous hyperplasia (AAH), adenocarcinoma in situ (AIS), minimally invasive adenocarcinoma (MIA), and invasive adenocarcinoma (IAC) on diagnostic CT images is important to evaluate the therapy options for lung cancer patients. In this work, we propose a joint deep learning model where the segmentation can better facilitate the classification of pulmonary GGNs. Based on our observation that masking the nodule to train the model results in better lesion classification, we propose to build a cascade architecture with both segmentation and classification networks. The segmentation model works as a trainable preprocessing module to provide the classification-guided ‘attention’ weight map to the raw CT data to achieve better diagnosis performance.

Multimodal deformable image registration is challenging but essential for many image-guided therapies, such as preoperative planning, intervention, and diagnosis. Recently, deep learning approaches has been extensively applied to medical image registration. Most networks adopt the mono-stream “high-to-low, low-to-high” fashion, which can achieve excellent recognition performance, but the localization accuracy, which is also important for predicting dense full-resolution deformation, will be deteriorated, especially for multimodal inputs with vast intensity differences. We have developed a novel unsupervised registration network, namely Full-Resolution Residual Registration Network (F3RNet), that can unite the strong capability of capturing deep representation for recognition with precise spatial localization of the anatomical structures by combining two paralleled processing streams in a residual learning fashion. One stream maintains reliable full-resolution information that guarantees precise adherence to voxel-level prediction. The other stream can fully learn the deep multi-scale residual representations to obtain robust recognition. Further, we factorize the 3D convolution to reduce the network parameters and enhance network efficiency.
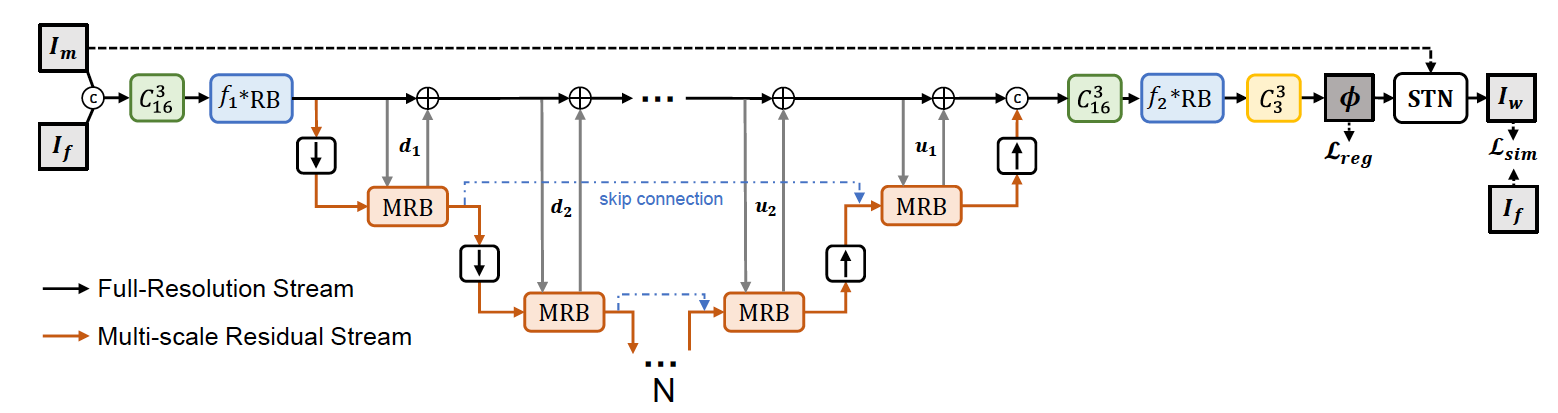